.avif)
Folding Data #38

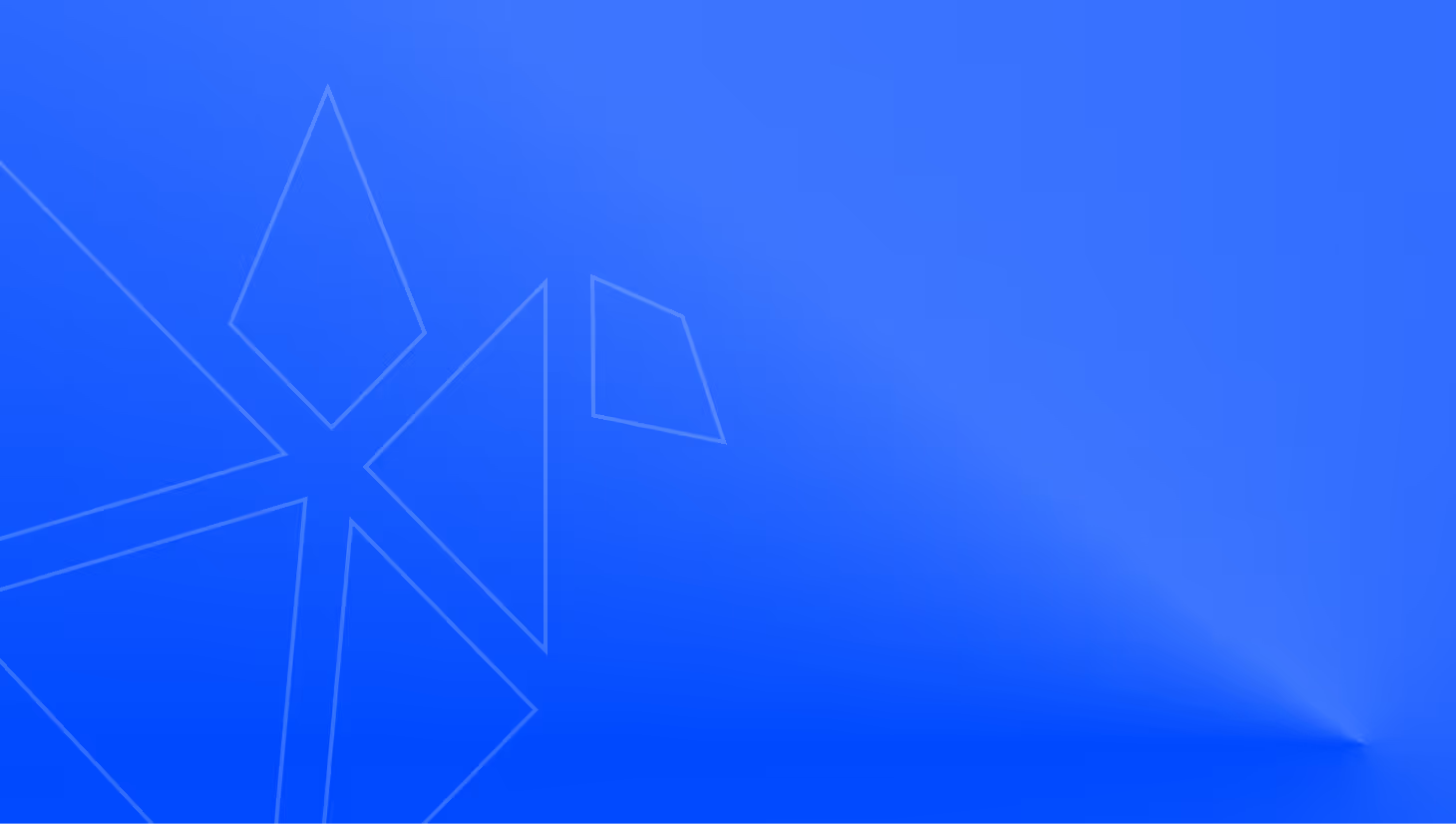
Oh, Airflow
I’ve written quite a bit about my love-hate relationship with Airflow, but Stephen Bailey said it all. Airflow enabled the impossible orchestration of data jobs spaghetti at high-growth data-hungry teams. But boy, how many sleepless nights I spent trying to write/fix/ship Airflow code. And shipping the Airflow code to production just to see if it works because there is no development environment... We should all be infinitely grateful to @mistercrunch and Airbnb who gave us Airflow but also I can’t wait for it to be disrupted into obsolescence by emerging technologies.
Stephen suggests that Astronomer may be the path to making Airflow hurt less but admitted in the comments that he chose to use Dagster. (I am trying Dagster Cloud this week, and so far, it seems very promising).
Will Astronomer, the main force behind Airflow these days, bring Airflow experience (not just hosting it but also using it) on par with present-day needs? Or will it follow the steps of Cloudera/Hortonworks in gradually getting sucked into the unappealing dead-end enterprise swamp?
Airflow is still so fundamental to the data stack that a discussion of the post on HackerNews quickly sprawled into fights on topics ranging from data democratization to data contracts. The thread has grown quiet – maybe someone should drop a couple of data mesh bombs to breathe new life into it.
The Airflow Problem
Tool of the week: MLU-EXPLAIN
https://mlu-explain.github.io/ – beautiful explanations of Machine Learning core concepts. From Amazon, of all places. It’s hard to become effective at ML without understanding the fundamentals, and I found it quite hard to master those through old thick textbooks. I think if more STEM education content is as well-designed, we'll see more great things happen in the world.
Machine Learning doesn't have to be hard
Before You Go
Apparently, Excel All-Star Battle is a pretty popular esport these days that gets streamed on ESPN . Don’t worry you can also watch it on YouTube.
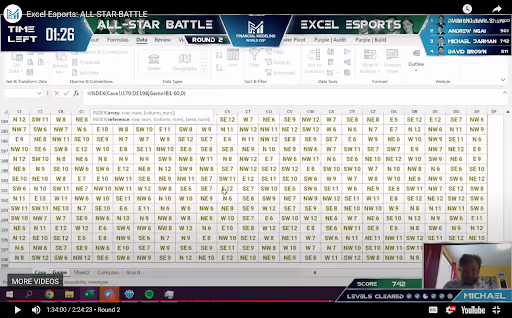
.avif)
