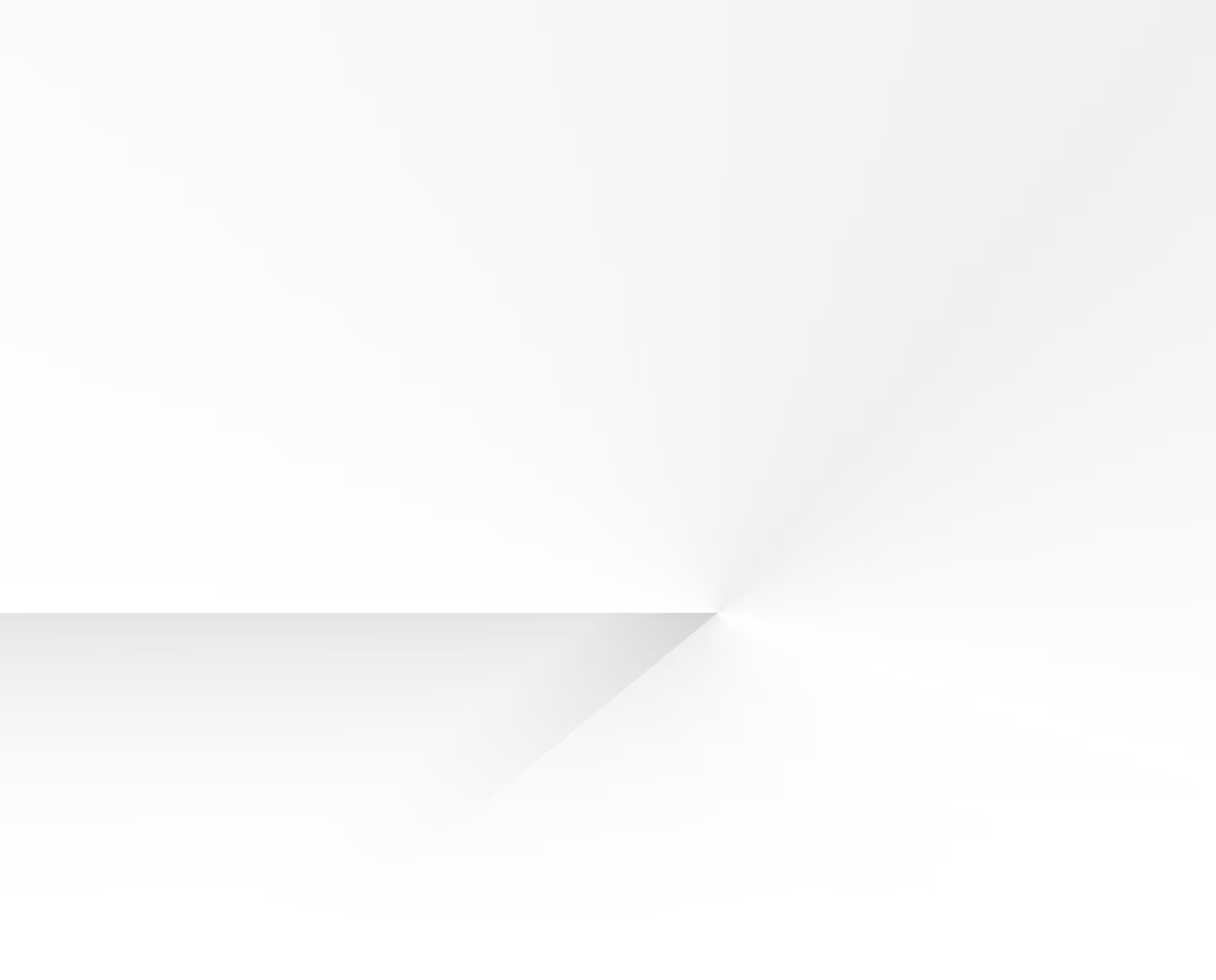
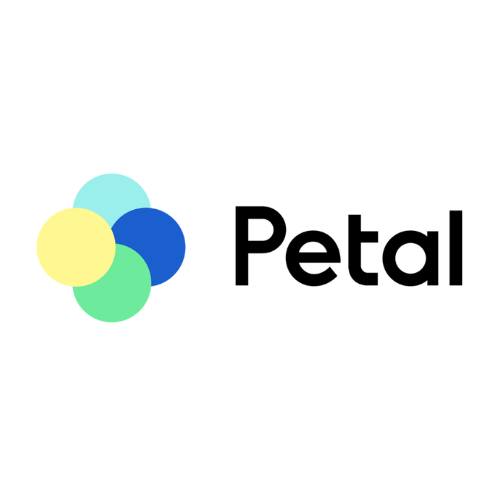
How Petal leveraged Datafold to transform its data quality practices
Data scientists know that their models are only as good as the data they rely on. And as data continuously changes, it is crucial to have best in class data integrity tools integrated in data pipelines. Using Datafold, Petal successfully enhanced data quality practices and improved the data science models powering its mission of democratizing credit access.
Introduction
Data scientists know that their models are only as good as the data they rely on. And as data continuously changes, it is crucial to have best in class data integrity tools integrated in data pipelines. Using Datafold, Petal successfully enhanced data quality practices and improved the data science models powering its mission of democratizing credit access.
We introduced Datafold initially thinking it would help fasten QA for Analytics and Data Infrastructure, and realized it was enabling many other teams to iterate faster and more accurately, including our Data Science and whole Engineering team. We plugged in Datafold in most of our development processes, as well as using it as the key tool to make sure all the data we receive is constant over time, which is a key requirement for a financial institution.
Data scientists know that their models are only as good as the data they rely on. And as data continuously changes, it is crucial to have best in class data integrity tools integrated in data pipelines. Using Datafold, Petal successfully enhanced data quality practices and improved the data science models powering its mission of democratizing credit access.
Petal
Petal is a fintech company that provides credit cards and financial services to underserved consumers. Its mission is to bring financial innovation and opportunity to everyone by using modern technology to help people build credit, avoid debt, and spend responsibly. Petal’s commitment to data quality ensures the precision of its data science models, which serve as the foundation for the company’s business decisions and strategies.
The challenge: Refactoring data science systems for improved data quality and performance
Petal's data science team wanted to leverage the dbt models originally designed for visualization and decision-making. However, they needed to avoid creating a situation where production data fed into analytics, which in turn informed data science models, leading to new data that could potentially influence production in a negative feedback loop.
Additionally, Petal's dbt models were complex, time-consuming to run, and were not designed for integration with Looker analytics dashboards. They needed to be overhauled to better serve end users.
As a fintech company, Petal’s operations are subject to strict regulatory requirements. Modifying the existing workflow required compliance with stringent standards for data integrity. This added layers of complexity and urgency to finding a sophisticated tool that fit easily into existing processes.
The solution: Building production data science workflows with confidence
Datafold enabled Petal to not only improve the data quality feedback loop between data science and engineering, but also helped accelerate the dbt model refactoring process. Using Datafold, Petal was able to speed up deployment cycles and improve performance of downstream assets, including Looker dashboards.
Automated QAs to improve production data quality
Prior to integrating Datafold, Petal’s analytics and data infrastructure teams relied heavily on manual SQL queries for QA testing. Whenever someone opened a PR, they needed to run a series of up to 15 manual SQL queries and add the output to double-check any unintentional data changes. Each PR required around 30 minutes of QA work, totaling about 15 hours per month spent validating their many datasets.
With Datafold's automated data testing, they significantly reduced the time spent on QA tasks. Instead of running custom SQL queries with each PR, teams could instead rely on Datafold’s bot to provide a high-level assessment of data discrepancies. They also could use the Datafold Cloud app for more granular information and understand what changed before merging anything to production. Petal's analytics and data infrastructure team was able to allocate their time more efficiently, focus on more strategic initiatives and reduce the burden of routine QA tasks.
Petal additionally used Datafold to seamlessly integrate third party data sources into their systems and compare existing and updated seed files in dbt before merging anything to production.
Refactoring complex data models with confidence
Datafold was also critical to speeding up the refactoring process and providing automated data validation. Petal innovatively broke down its large data models into smaller components and compared them using Data Diff. This enabled refactoring models incrementally and addressing discrepancies and errors at each stage to ensure integrity.
The result: A streamlined data workflow
Petal's strategic overhaul of its existing data workflow using Datafold made it easy to achieve high data quality standards for data science projects and improve all decision-making processes across the company. Datafold helped provide much-needed visibility to avoid unwanted data changes and was a powerful tool to accelerate the refactoring process for better BI performance.
Improved collaboration between data science and engineering
Datafold improved collaboration between data scientists and analysts by providing a structured framework for data validation and quality assurance. Before using Datafold, the team used ad hoc and manual queries to verify the quality of their data, which was time-consuming and labor-intensive, especially considering the frequency of their updates (approximately 30 PRs a month). Using Data Diffs in their CI process helped automate data validation throughout the data pipeline and increase transparency of data quality between the two teams.
Eliminated data quality incidents entirely
Since Petal implemented Datafold Cloud in 2022, Petal has not experienced a single major data quality incident. Datafold’s automated data quality testing system is helping to maintain high data quality standards and integrity, which is critical to Petal’s success.